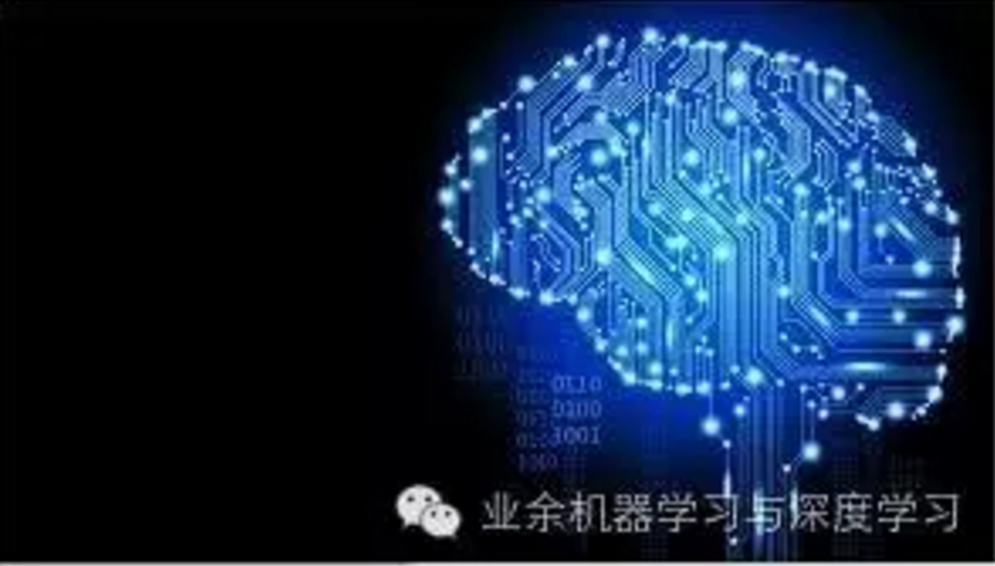
Deep ADMM-Net for Compressive Sensing MRI
Yan Yang, Xi'an Jiaotong University; Jian Sun*, Xi'an Jiaotong University; Huibin Li, ; Zongben Xu,
Swapout: Learning an ensemble of deep architectures
Saurabh Singh*, UIUC; Derek Hoiem, UIUC; David Forsyth, UIUC
Deep Learning without Poor Local Minima
Kenji Kawaguchi*, MIT
A Powerful Generative Model Using Random Weights for the Deep Image Representation
Kun He, Huazhong University of Science and Technology; Yan Wang*, HUAZHONG UNIVERSITY OF SCIENCE; John Hopcroft, Cornell University
Generating Images with Perceptual Similarity Metrics based on Deep Networks
Alexey Dosovitskiy*, ; Thomas Brox, University of Freiburg
Deep Alternative Neural Networks: Exploring Contexts as Early as Possible for Action Recognition
Jinzhuo Wang*, PKU; Wenmin Wang, peking university; xiongtao Chen, peking university; Ronggang Wang, peking university; Wen Gao, peking university
Proximal Deep Structured Models
Shenlong Wang*, University of Toronto; Sanja Fidler, ; Raquel Urtasun,
Weight Normalization: A Simple Reparameterization to Accelerate Training of Deep Neural Networks
Tim Salimans*, ; Diederik Kingma,
Direct Feedback Alignment Provides Learning In Deep Neural Networks
Arild Nøkland*, None
How Deep is the Feature Analysis underlying Rapid Visual Categorization?
Sven Eberhardt*, Brown University; Jonah Cader, Brown University; Thomas Serre,
Regularization With Stochastic Transformations and Perturbations for Deep Semi-Supervised Learning
Mehdi Sajjadi*, University of Utah; Mehran Javanmardi, University of Utah; Tolga Tasdizen, University of Utah
Local Similarity-Aware Deep Feature Embedding
Chen Huang*, Chinese University of HongKong; Chen Change Loy, The Chinese University of HK; Xiaoou Tang, The Chinese University of Hong Kong
Deep Learning Models of the Retinal Response to Natural Scenes
Lane McIntosh*, Stanford University; Niru Maheswaranathan, Stanford University; Aran Nayebi, Stanford University; Surya Ganguli, Stanford; Stephen Baccus, Stanford University
Deep Learning Games
Dale Schuurmans*, ; Martin Zinkevich, Google
Improved Deep Metric Learning with Multi-class N-pair Loss Objective
Kihyuk Sohn*,
Stacked Approximated Regression Machine: A Simple Deep Learning Approach
Zhangyang Wang*, UIUC; Shiyu Chang, UIUC; Qing Ling, USTC; Shuai Huang, UW; Xia Hu, ; Honghui Shi, UIUC; Thomas Huang, UIUC
Learning Structured Sparsity in Deep Neural Networks
Wei Wen*, University of Pittsburgh; Chunpeng Wu, University of Pittsburgh; Yandan Wang, University of Pittsburgh; Yiran Chen, University of Pittsburgh; Hai Li, University of Pittsburg
Stochastic Multiple Choice Learning for Training Diverse Deep Ensembles
Stefan Lee*, Indiana University; Senthil Purushwalkam, Carnegie Mellon; Michael Cogswell, Virginia Tech; Viresh Ranjan, Virginia Tech; David Crandall, Indiana University; Dhruv Batra,
Learning to Communicate with Deep Multi-Agent Reinforcement Learning
Jakob Foerster*, University of Oxford; Yannis Assael, University of Oxford; Nando de Freitas, University of Oxford; Shimon Whiteson,
DeepMath - Deep Sequence Models for Premise Selection
Geoffrey Irving*, ; Christian Szegedy, ; Alexander Alemi, Google; Francois Chollet, ; Josef Urban, Czech Technical University in Prague
Toward Deeper Understanding of Neural Networks: The Power of Initialization and a Dual View on Expressivity
Amit Daniely*, ; Roy Frostig, Stanford University; Yoram Singer, Google
Learning the Number of Neurons in Deep Networks
Jose Alvarez*, NICTA; Mathieu Salzmann, EPFL
Variational Autoencoder for Deep Learning of Images, Labels and Captions
Yunchen Pu*, Duke University; Zhe Gan, Duke; Ricardo Henao, ; Xin Yuan, Bell Labs; chunyuan Li, Duke; Andrew Stevens, Duke University; Lawrence Carin,
Deep Learning for Predicting Human Strategic Behavior
Jason Hartford*, University of British Columbia; Kevin Leyton-Brown, ; James Wright, University of British Columbia
Improved Dropout for Shallow and Deep Learning
Zhe Li, The University of Iowa; Boqing Gong, University of Central Florida; Tianbao Yang*, University of Iowa
A Probabilistic Framework for Deep Learning
Ankit Patel, Baylor College of Medicine; Rice University; Tan Nguyen*, Rice University; Richard Baraniuk,
Stochastic Variational Deep Kernel Learning
Andrew Wilson*, Carnegie Mellon University; Zhiting Hu, Carnegie Mellon University; Ruslan Salakhutdinov, University of Toronto; Eric Xing, Carnegie Mellon University
Deep Neural Networks with Inexact Matching for Person Re-Identification
Arulkumar Subramaniam, IIT Madras; Moitreya Chatterjee*, IIT Madras; Anurag Mittal, IIT Madras
Image Restoration Using Very Deep Convolutional Encoder-Decoder Networks with Symmetric Skip Connections
Xiao-Jiao Mao, Nanjing University; Chunhua Shen*, ; Yu-Bin Yang,
Exponential expressivity in deep neural networks through transient chaos
Ben Poole*, Stanford University; Subhaneil Lahiri, Stanford University; Maithra Raghu, Cornell University; Jascha Sohl-Dickstein, ; Surya Ganguli, Stanford
Synthesizing the preferred inputs for neurons in neural networks via deep generator networks
Anh Nguyen*, University of Wyoming; Alexey Dosovitskiy, ; Jason Yosinski, Cornell; Thomas Brox, University of Freiburg; Jeff Clune,
Deep Submodular Functions
Brian Dolhansky*, University of Washington; Jeff Bilmes, University of Washington, Seattle
Hierarchical Deep Reinforcement Learning: Integrating Temporal Abstraction and Intrinsic Motivation
Tejas Kulkarni, MIT; Karthik Narasimhan*, MIT; Ardavan Saeedi, MIT; Joshua Tenenbaum,
Deep Unsupervised Exemplar Learning
MIGUEL BAUTISTA*, HEIDELBERG UNIVERSITY; Artsiom Sanakoyeu, Heidelberg University; Ekaterina Tikhoncheva, Heidelberg University; Björn Ommer,
Deep Exploration via Bootstrapped DQN
Ian Osband*, DeepMind; Charles Blundell, DeepMind; Alexander Pritzel, ; Benjamin Van Roy,
Learning Deep Embeddings with Histogram Loss
Evgeniya Ustinova, Skoltech; Victor Lempitsky*,
Maximal Sparsity with Deep Networks?
Bo Xin*, Peking University; Yizhou Wang, Peking University; Wen Gao, peking university; David Wipf,
Tagger: Deep Unsupervised Perceptual Grouping
Klaus Greff*, IDSIA; Antti Rasmus, The Curious AI Company; Mathias Berglund, The Curious AI Company; Tele Hao, The Curious AI Company; Harri Valpola, The Curious AI Company
Learning Deep Parsimonious Representations
Renjie Liao*, UofT; Alexander Schwing, ; Rich Zemel, ; Raquel Urtasun,
Optimal Architectures in a Solvable Model of Deep Networks
Jonathan Kadmon*, Hebrew University; Haim Sompolinsky
An Architecture for Deep, Hierarchical Generative Models
Philip Bachman*,
Understanding the Effective Receptive Field in Deep Convolutional Neural Networks
Wenjie Luo*, University of Toronto; Yujia Li, University of Toronto; Raquel Urtasun, ; Rich Zemel,
Disentangling factors of variation in deep representation using adversarial training
Michael Mathieu, NYU; Junbo Zhao, NYU; Aditya Ramesh, NYU; Pablo Sprechmann*, ; Yann LeCun, NYU